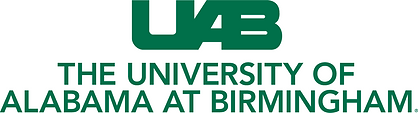
ZHANG LAB'S WEBSITE
This site is under draft
Latest draft: Sep 2 2022
Bioinformatics, Big Data, and Artificial Intelligence in Cardiovascular Research
The heart is a heterogeneous organ composed of numerous cell types, and even cells of the same lineage likely respond differently to myocardial injury. This heterogeneity can be accommodated by single-cell and single-nucleus RNA sequencing (scRNAseq and snRNAseq, respectively), but because the resulting dataset includes expression information for thousands of individual genes in thousands of individual cells, its dimensionality is immense. Thus, the results of these analyses can only be interpreted via the application of state-of-the-art big-data bioinformatics techniques. Yet, these techniques still find it difficult to identify important signaling regulators and specific cardiomyocytes associated with regeneration after injury, which is among the key challenges in cardiovascular research.
In UAB Zhang lab, we have made significant efforts in customizing Artificial Intelligence (AI) techniques to analyze scRNAseq data from the regenerative hearts. Drs. Thanh Nguyen, Yuji Nakada, Ms. Yuhua Wei and others have developed a new AI-based pipeline to analyze cardiac scRNAseq data. The pipeline includes i) Autoencoder to separate cardiomyocytes and identify cardiomyocyte subpopulations; ii) sparse analysis to quantify the cell-cycle, signaling pathways, and other biological processes among the regenerative-heart cardiomyocytes; and iii) semi-supervised learning to infer the transformation of the highly-proliferative cardiomyocyte subpopulations following a heart injury. The pipeline finds a new cardiomyocyte cluster, called 'CM1', exclusive for the regenerative hearts that underwent Apical Resection on postnatal day 1 (ARP1). This 'CM1' cluster co-upregulates T-box transcription factors 5 and 20 (TBX5 and TBX20, respectively), Erb-B2 receptor tyrosine kinase 4 (ERBB4), and G Protein-Coupled Receptor Kinase 5 (GRK5), as well as genes associated with the proliferation and growth of cardiac muscle. Furthermore, this cluster still presents 4 weeks after ARP1 injury, which might contribute to the remuscularization following the second myocardial infarction on postnatal day 28. Other state-of-the-art scRNAseq pipelines, including Seurat and ScanPY, could not identify nor characterize the 'CM1' cluster.
Upon these successes, our lab plans to mature the AI pipeline in the following aspects. First, The computing resources required by Autoencoder will be reduced so that the technique can run efficiently using a 12-64GB Graphic Processing Unit. Second, the Autoencoder can be reused in different cardiac scRNAseq data, which will eliminate a large amount of time spent to compute the Autoencoder from the beginning. Third, a new user-friendly and easy-to-use version of the pipeline will be implemented so that a large research community can widely use the pipeline.
Read more:
- Nguyen, T.M., Wei, Y., Nakada, Y., Zhou, Y. and Zhang, J., Cardiomyocyte cell-cycle regulation in neonatal large mammals: Single Nucleus RNA-sequencing Data analysis via an Artificial-intelligence–based pipeline. Frontiers in Bioengineering and Biotechnology, p.972.
- Nakada, Y., Zhou, Y., Gong, W., Zhang, E.Y., Skie, E., Nguyen, T., Wei, Y., Zhao, M., Chen, W., Sun, J. and Raza, S.N., 2022. Single Nucleus Transcriptomics: Apical Resection in Newborn Pigs Extends the Time Window of Cardiomyocyte Proliferation and Myocardial Regeneration. Circulation, 145(23), pp.1744-1747.
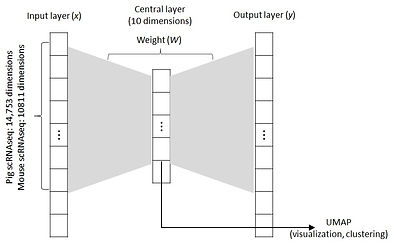
The AI-based Autoencoder architecture. The Autoencoder algorithm is a deep-neural-network AI technique used to synthesize, denoise, or translate data The autoencoding procedure is performed by alternately encoding the input layer into the embedded layer and decoding the embedded layer into the output layer until the output layer matches the input layer with maximum fidelity. Then, the embedded layer is considered an accurate low-dimensional representation of the input data .
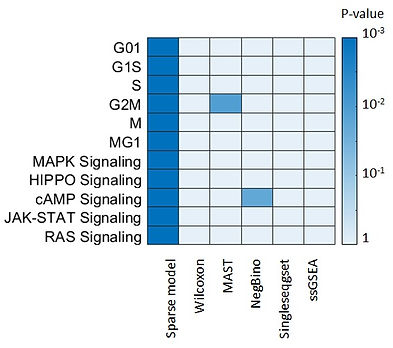
Only Sparse Model can identify cell cycle (G01, G1S, S, G2M, M, MG1) and signaling pathways (MAPK, HIPPO, cAMP, JAK-STAT, RAS) associated with regenerative-heart cardiomyocyte. Here, the P-value produced by each method sparse model, Wilcoxon ranksum test, MAST, Negative Binomial (NegBino) test, Singleseqset, and ssGSEA) is used to indicated whether a cell cycle phase or signaling pathway is associated with regeneration. Smaller P-value implies stronger association, and P-value < 0.05 is considered significant.